BLOG | GENERATIVE AI
Understanding Artificial General Intelligence (AGI)
Artificial general intelligence (AGI), also known as strong AI, has long been a holy grail for AI researchers. AGI refers to a machine with the capacity for learning and intelligence that matches or exceeds that of a human across a wide range of cognitive tasks. Although narrow AI has achieved superhuman performance in specialized domains like chess, go, and image recognition, developing systems with more generalized learning capabilities remains an open challenge.
In this technology guide, we provide an overview of the current state of AGI research, major approaches, key players, and critical issues still to be addressed on the path toward human-level machine intelligence.
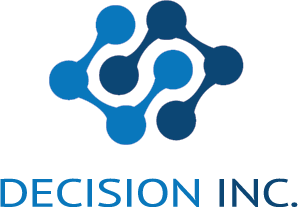
What is Artificial General Intelligence?
Artificial general intelligence (AGI) refers to machines that can learn and reason across a wide range of domains, similar to human intelligence. Unlike narrow AI systems designed for specific tasks, AGI aims for more generalized problem-solving abilities. Think of AGI as a computer that could perform any intellectual task a human could, from analysing financial data to providing healthcare advice.
While we do not yet have human-level AGI, researchers are actively exploring potential paths forward. Current state-of-the-art AI systems display specialized intelligence focused on perception, language processing, game playing, and more. AGI remains on the horizon as an aspirational goal for many in the field.
The hallmarks of AGI include:
- Learning a wide variety of tasks and skills rather than specializing in a single domain
- Transferring knowledge between different problems and situations
- Understanding and responding appropriately to complex, nuanced inputs
- Reasoning about abstract concepts and using logic and creativity to solve novel problems
AGI systems do not yet exist, but developing prototypes with increasing autonomy and general applicability to diverse tasks remains an active goal for leading AI research institutes and companies.
Why Does AGI Matter for Business Leaders?
As AI capabilities continue advancing rapidly, business leaders should understand the potential impacts and implications of future AGI:Â
- It could enable systems that adapt quickly across diverse situations, reducing costs. Rather than developing separate AI for different business functions, AGI could provide flexible solutions.
- It may automate certain high-level roles over time. While routine tasks have already been automated, AGI could substitute human roles involving strategy, planning, and creative responsibilities.
- It can raise complex ethical questions around transparency, bias, control, and more. As systems become more autonomous, responsible governance becomes critical.
In summary, while promising great economic potential, AGI introduces new uncertainties and risks that executives should monitor and prepare for.
What is the Difference Between Artificial Intelligence and Artificial General Intelligence?
Artificial Intelligence (AI) refers broadly to any system that can perceive its environment and take actions to maximize its chances of achieving specified goals. This encompasses a wide range of technologies from simple rule-based algorithms to sophisticated deep learning models.
In contrast, Artificial General Intelligence (AGI) aims to build systems with more generalized problem-solving capabilities that can handle unfamiliar situations. While specialized AI focuses on excelling in a single domain, AGI targets the flexibility and adaptability seen in human cognition.
To illustrate the difference, consider machine translation services. AI-powered translation tools utilize vast datasets and complex neural networks to convert text between languages with impressive accuracy. However, the system only understands language and lacks real-world common sense or reasoning skills. It cannot answer questions about what was translated or summarize key points.
An AGI assistant by contrast could not just translate, but comprehend the content, draw connections to relevant concepts, identify possible implications for the user, and support further dialogue. This ability to link knowledge across domains and skills is a key distinction of artificial general intelligence.
Leaders should recognize that while narrow AI drives many current business benefits, true AGI promises (or threatens) much deeper potential disruption across every industry. Monitoring this boundary between specialized and general systems remains crucial.
Approaches to Achieving AGI
There are several distinct approaches researchers are taking to try to achieve AGI, including:
- Whole brain emulation: Attempting to directly replicate the structures and pathways of the human brain in silicon. This could allow an AGI system to leverage the problem-solving capabilities evolution has produced in the brain.
- Integrated cognitive architectures: Constructing unified computational models that combine perception, reasoning, learning, problem solving, and decision making capabilities.
- Self-supervised learning and scalable machine learning: Allowing AI models to learn broadly applicable representations from exposure to massive datasets, without extensive human labeling or supervision.
- Hybrid systems: Combining neural networks with classical symbolic AI to integrate logical reasoning abilities with pattern recognition strengths.
There are merits to each approach, but major breakthroughs will likely require integrating multiple methods tailored to different aspects of intelligence. No consensus front-runner methodology has yet emerged.
Critical Challenges on the Road to AGI
Despite promising advancements, many fundamental challenges remain to realise fully general human-level AI, including:
Common sense reasoning – Encoding the vast stores of contextual common sense knowledge humans accumulate through experience to allow sound judgment.
Self-supervised learning – Developing systems that can direct their own learning by setting goals, asking questions, designing experiments and simulations.
Explainable model behaviors – Engineering transparency so humans can understand the reasoning behind AGI system actions and ensure alignment with human values.
Validation and control – Creating rigorous testing protocols for complex behaviors and building in oversight mechanisms without limiting performance.
AGI research remains squarely focused on advancing machine autonomy and intelligence rather than developing commercial applications in the near term.
But success in this grand challenge could unlock a new era of possibility in terms of scientific discoveries, business innovations, and enhancement of human potential.
What is the Current Progress Toward AGI?
Although we do not have human-level AGI yet, active research areas include:
Integrating separate AI capabilities – Combining perception, language, planning, and learning systems to enable more generalised skills.
Architecture exploration – Testing different computational frameworks to support reasoning, knowledge representation, and decision making.
Simulated environments – Using virtual worlds to provide safe, rapid testing environments for AGIs before real-world interaction.
Neuroscience inspiration – Drawing from our understanding of natural intelligence in the brain to guide AGI architectures.
Benchmarking – Establishing rigorous testing suites to measure and track AGI progress across different capabilities.
While many hard problems remain, AGI research leverages existing AI advances and continues steadily, if slowly. Leaders should track technical milestones through reputable technology research firms.
What is a Realistic Timeline for AGI Emergence?
Expert opinions diverge greatly on when we will develop AGIs with abilities rivaling humans. Predictions range from 10-15 years on the aggressive end to over 50 years for the more conservative outlook. The truth likely lies somewhere in the middle.
As with any complex technology forecasting, uncertainty remains high. Unexpected breakthroughs or barriers could accelerate or delay timelines.
Regardless, business leaders should monitor incremental progress rather than wait for definitive AGI arrival before planning.
Prudent steps today include:
- Discussing potential scenarios and strategic responses
- Evaluating value chain impacts
- Developing governance frameworks and ethics policies
- Prototyping applications for early capability testing
- Tracking relevant startups and research directions
While the AGI future remains unclear, leaders who understand the landscape and implications can prepare their organisations for success.
Read More
AI-driven solution enables deeper fan insights and automates qualitative feedback
Decision Inc. Australia's AI-driven solution enables deeper fan insights and automates qualitative feedbackSolution OverviewOne of Australia’s leading sporting organisations, comprising men’s, women’s, and e-sports competitions, is the peak body of one of the world’s...
Major Hospitality Group Customer Feedback Review Process Revitalised with Automation
Decision Inc. Australia Automates Customer Feedback Review Process and Leverages AI to Streamline Sentiment Analysis for Major Hospitality GroupSolution OverviewAn Australian hospitality group, which operates cinemas, hotels, restaurants and resorts across Australia,...
Watterson Intelligent Automation Case Study
Decision Inc. Australia Streamlines Media Analysis and Sentiment, Leveraging AI and Automation for WattersonSolution OverviewWatterson is a boutique public relations and marketing communications consultancy.New South Wales, AustraliaMedia &...